Connect AI to External Systems - Model Context Protocol
You’ve learned how to build agentic workflows and autonomous agents capable of complex reasoning and planning. However, for these AI systems to be truly effective in real-world scenarios, they need interact reliably with external systems - databases, APIs, file systems, and various specialized tools. Connecting these components individually often leads to brittle, hard-to-maintain integrations, creating a significant bottleneck known as the “M x N” problem where every new AI application might require custom code for every tool it needs.
This tutorial introduces the Model Context Protocol (MCP)1, an open standard designed to solve this integration chaos. We will move beyond theoretical concepts and dive into practical implementation. You will build both an MCP server, acting as a bridge to a simple task management system, and an MCP client integrated into a Streamlit application, allowing a local LLM (via Ollama) to manage a todo list by communicating through the standardized MCP interface.
Tutorial Goals
- Understand what is the Model Context Protocol (MCP)
- Implement a basic MCP server exposing custom tools
- Build an MCP client to connect and interact with the server
- Integrate MCP tools into an LLM-powered agent
- Develop a Streamlit app for interacting with the MCP-enabled agent
- Manage a simple application (todo list) through MCP interactions
What is Model Context Protocol (MCP)?
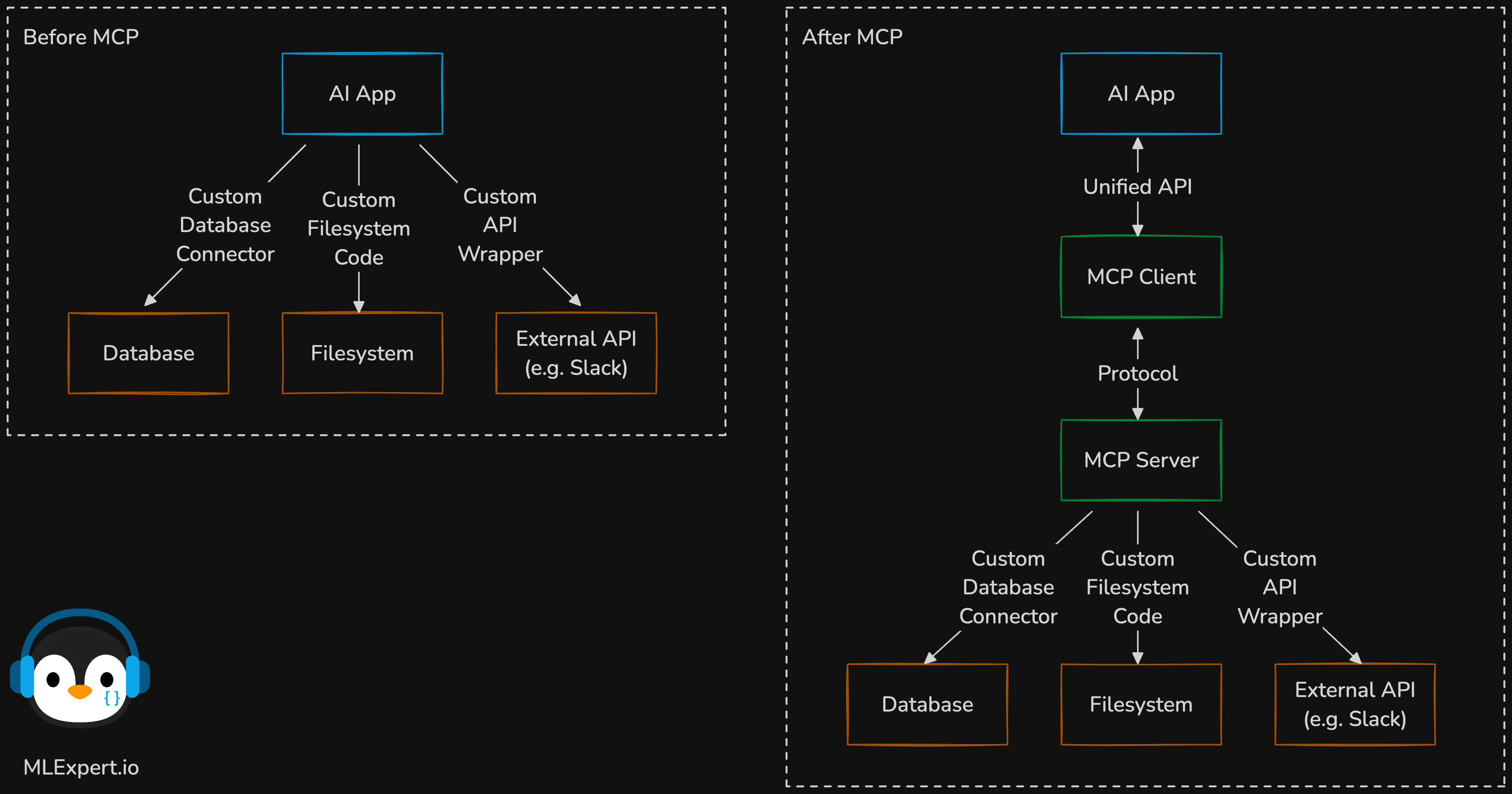